Deep learning-based smart camera applications in manual assembly
Manual assembly tasks are error prone. Deep learning-based smart cameras can be used to recognize assembly tasks, allowing to provide operators with live feedback and instructions to reduce error rates. The use of synthetic training data thereby allows to simplify the training process for new or adapted deep learning models.
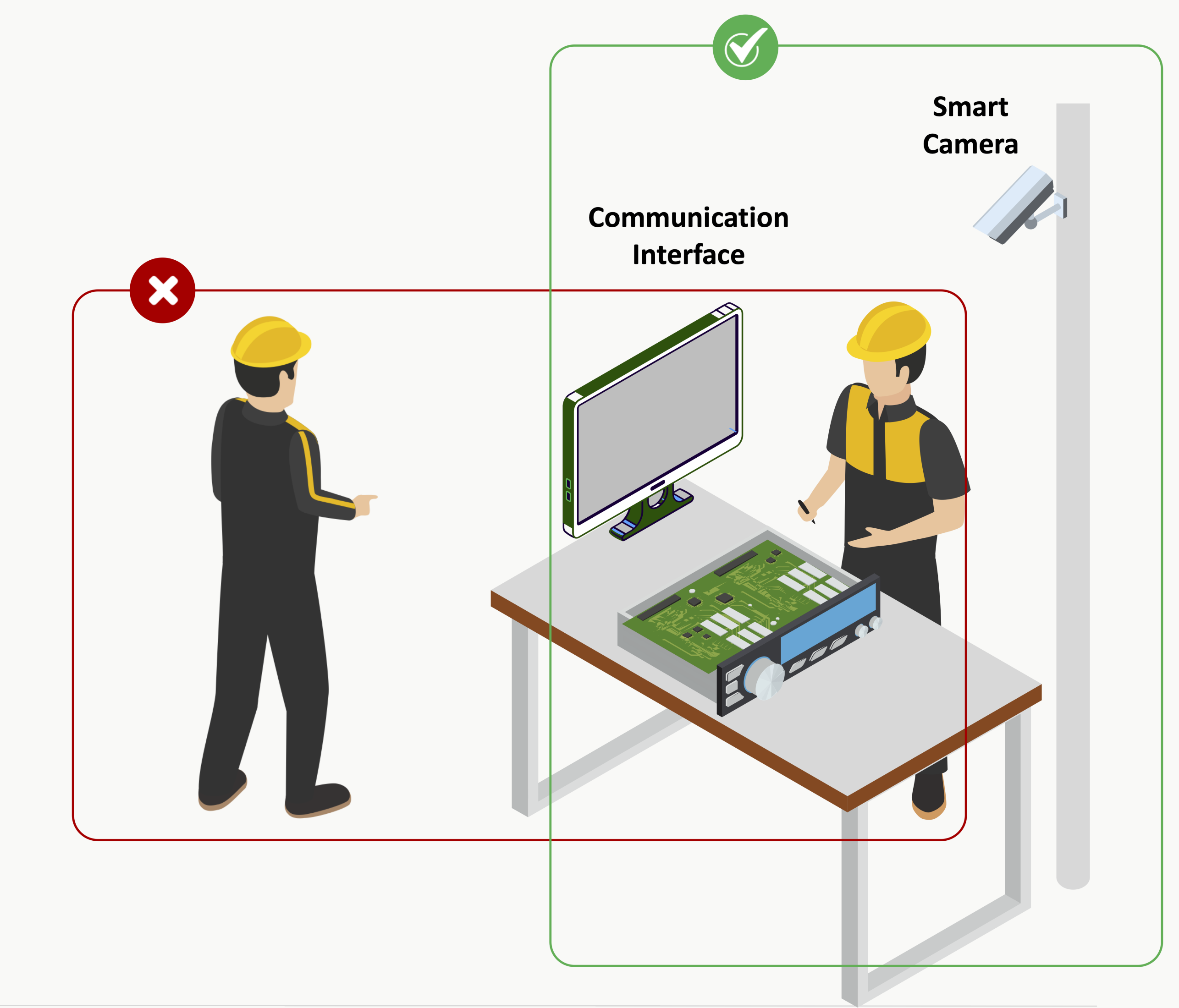
Automated recognition of manual assembly tasks
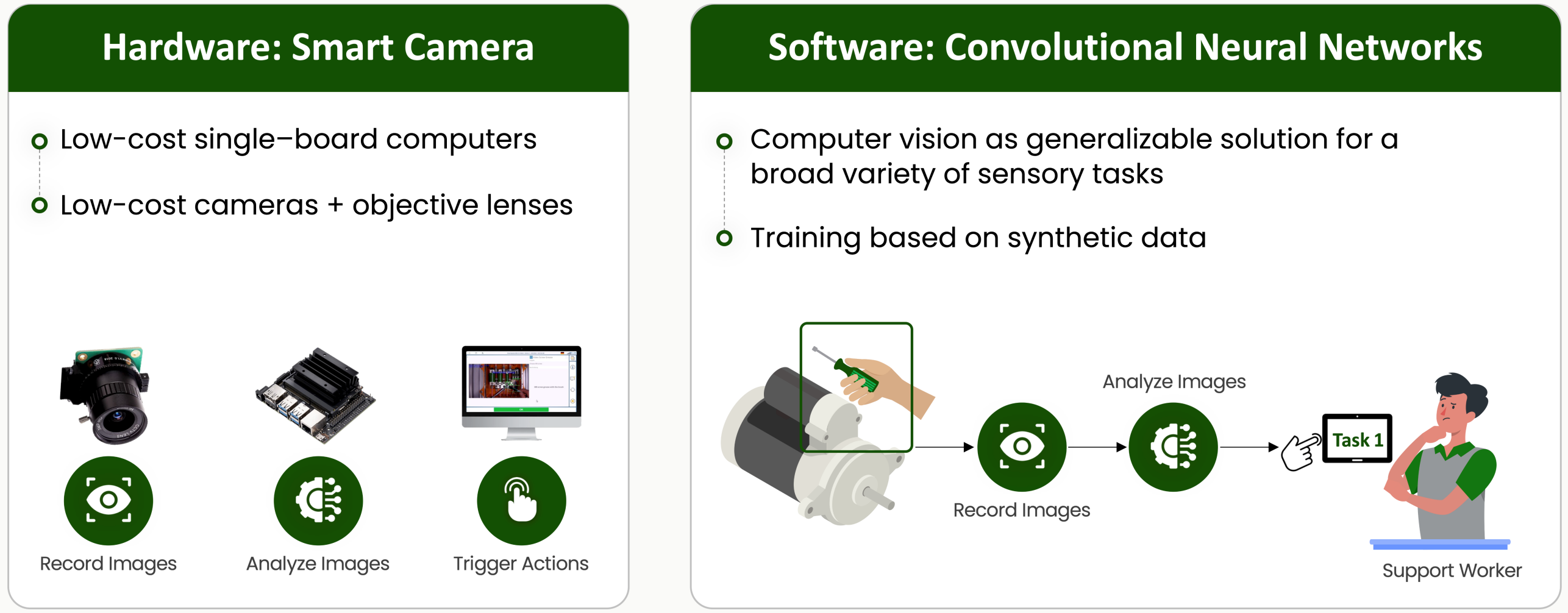
Deep learning-based computer vision models can be used to automatically analyze assembly tasks. If specific tasks can be recognized and distinguished from others, their realization can be documented, and operators can be provided with feedback about their realization or instructions for upcoming tasks.
In our research, we implement and analyze different approaches for state- and action-recognition in manual assembly specifically. For this, we collaborate with industrial partners, allowing the analysis of new computer vision approaches in live assembly lines.
Our most recent project was realized together with Bossard AG and Huber+Suhner AG. The project is summarized in the following video.
Synthetic training data for smart cameras
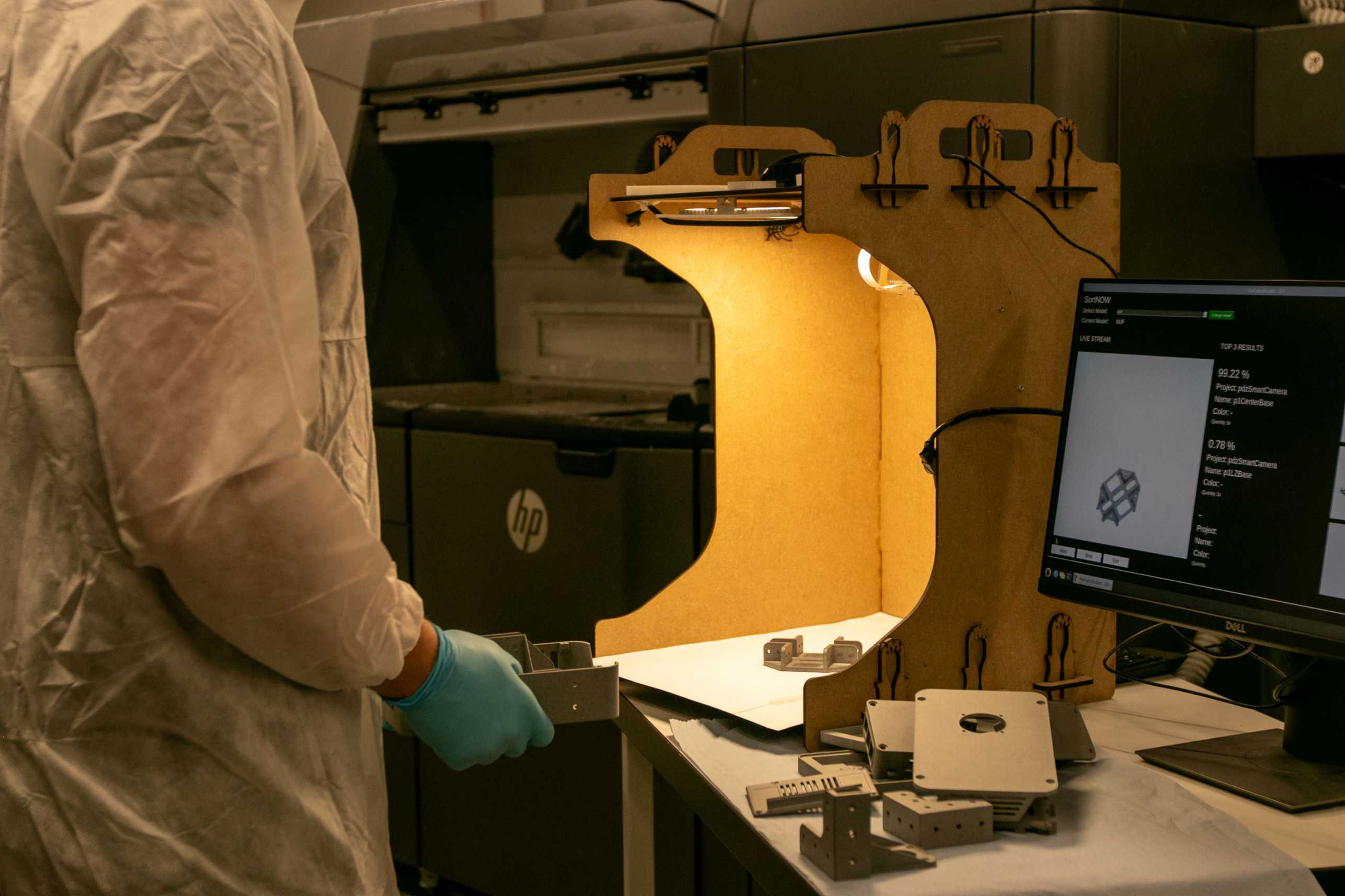
One hurdle for the industrial application of deep learning-based computer vision models is the availability of training data.
A way to tackle this hurdle is the generation of synthetic training data: Computer vision models can be trained solely on renderings created on the base of CAD data, which allows for full automation of the training data generation and model training process. This was showcased in the application scenario of AM part sorting, where parts are recognized manually. In a fully automated pipeline, deep learning models were trained with the purpose of recognizing AM parts after printing external page [1].
Synthetic training data for error recognition in manual assembly
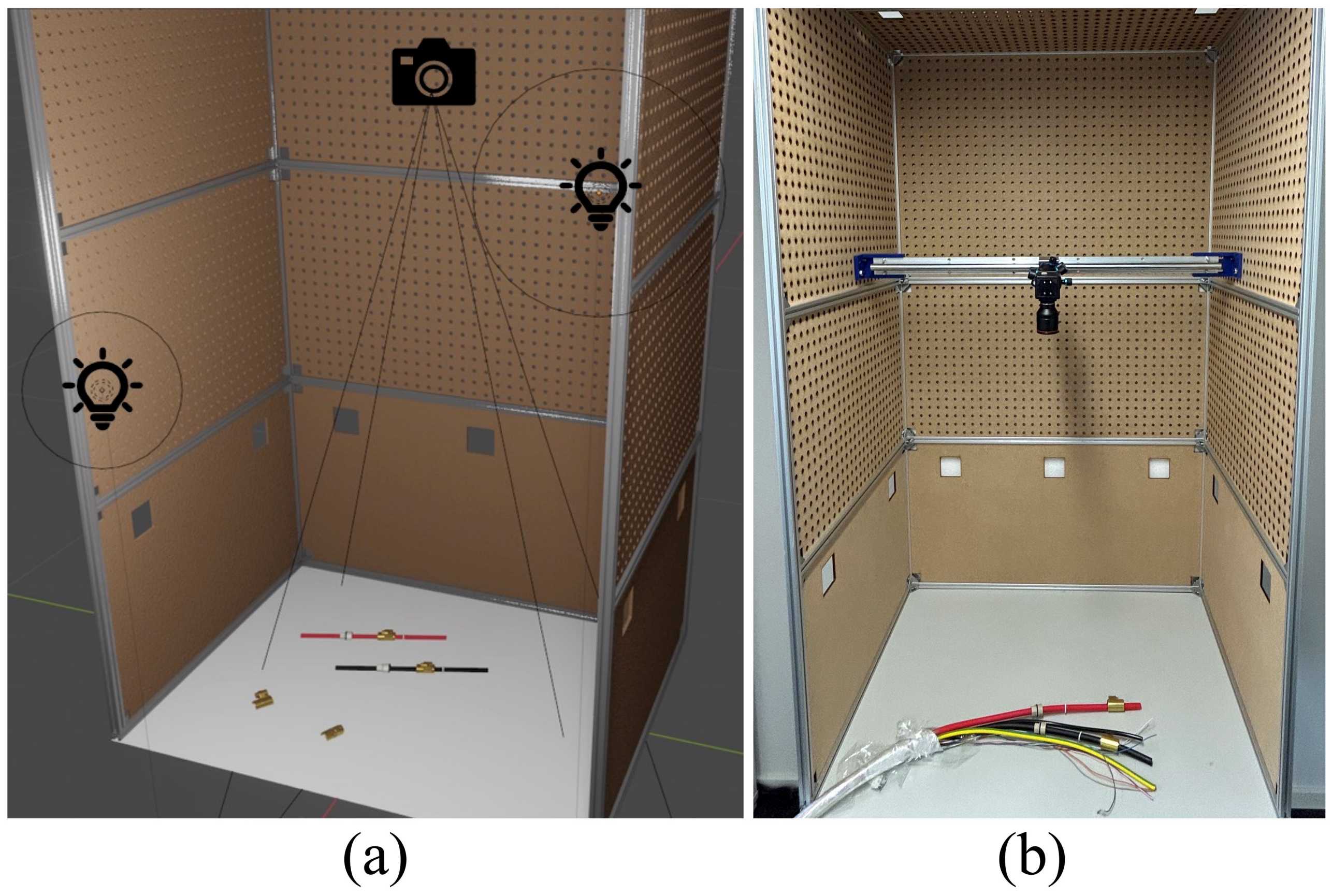
Deep learning-based smart cameras trained on synthetic data can be used to recognize different assembly states. If the assembly scenario is adapted, for example with the exchange of a component, a new deep learning model can be trained automatically. No data recording or manual labelling is required due to the use of synthetic training data.
The approach was demonstrated in the application scenario of a cable assembly sequence. With the use of deep learning models solely trained on synthetic data, different error states can be automatically recognized, and workers can be alerted to prevent rework.
For more information please contact Jonas Conrad or Julian Ferchow.